By Brian Wu, The Edge Singapore
Aggregate Asset Management’s (AAM) investment philosophy is reflected in its name — Aggregate. Its funds such as the Aggregate Value Fund (AVF) and the Aggregate Global Equities Fund (AGEF) are aggregations of many stocks. For instance, AVF comprises some 1,500 stocks, and AGEF around 500 stocks. Their resilience, despite a rocky 2022 — against a backdrop of war, famine, inflation, climate change and an outsized dependence on fossil fuels by the global economy — is due to their diversified portfolios.
Based on AAM’s proprietary machine learning outcomes, the funds invest in the top 1% of stocks in the investing universe. Among the metrics used from the machine learning model is R&D expenses as a percentage of market capitalisation. AAM’s fund managers place weight on value investing metrics as well, which are also incorporated in AAM’s proprietary machine learning models.
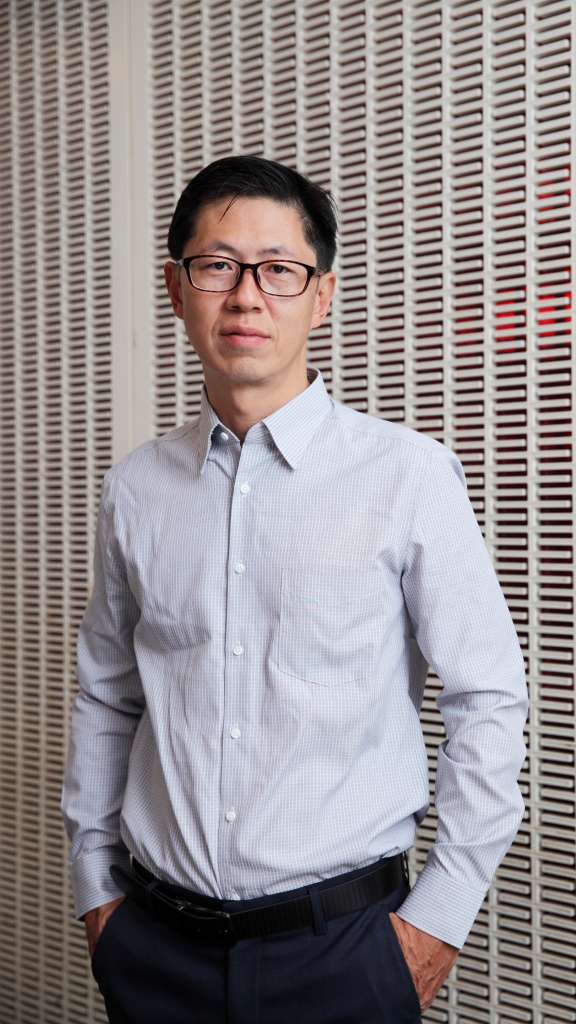
Speaking at a webinar hosted by The Edge Singapore in partnership with AAM, titled “Managing Global Uncertainties; Seizing Global Opportunities”, executive director of AAM Eric Kong says that while the convoluted geopolitical backdrop of the global economy could understandably put off investors, he believes that investors should not be “fearful” of market panics and stick to sound investment plans.
Kong describes a “perpetual energy machine” that can be achieved through investing in equities across global markets and sectors. Although perpetual motion is a concept that violates laws of thermodynamics, Kong believes this analogy is the fundamental basis of wealth generation.
According to Kong, there is a two-step process to building a robust portfolio akin to this “perpetual energy machine”. The first involves investing in multiple countries, starting with a few and building up to what research points to as the “optimal” number of 20. “You don’t want to invest in just a single country because you can’t predict what happens to individual countries,” he says.
He highlights time periods in which countries experienced negative growth. Singapore’s own Straits Times Index, for example, has “gone nowhere”. The index peaked at around 3,800 points in 2008 just before the Global Financial Crisis. More than a dozen years on, it has not gone quite back near that level, managing at round 3,100 points now. As such, being invested in multiple countries can “buffer” negative growth in individual markets to earn portfolio gains, reasons Kong.
Next, multiple equally-weighted stocks in each country need to be added for diversification. Investing in indexes is not necessarily a good way to achieve this, since indexes are usually heavily weighted in favour of larger companies. “If you were to buy the MSCI Korea ETF, a quarter of your portfolio would go towards Samsung Electronics. In Taiwan, a quarter of your money again goes into Taiwan Semiconductor Manufacturing Company,” he points out.
Quoting Hari Seldon, a fictional character from Isaac Asimov’s Foundation television series, Kong says: “You can’t predict the movement of an individual company, but you can predict the general direction of a group of companies.”
By purchasing hundreds of stocks across multiple countries at almost equal weightings, he believes that this “grand design” is essentially “indestructible”, hedging against unforeseeable market events.
Over the last nine years, AAM has delivered a compound annual growth rate of 8% in returns for its investors. The firm now manages a total of $600 million, with more than 1,000 clients, who each invest at least $100,000 with the fund.
Demystifying machine learning
But AAM goes even further in its efforts towards diversification. Harry Huo, head of special projects at AAM, says that that in the face of further “cataclysmic changes”, such as the crises the world has experienced in the last two years, a source of reliance could come in the form of machine learning, a subset of artificial intelligence (AI) which he hopes to “demystify”.
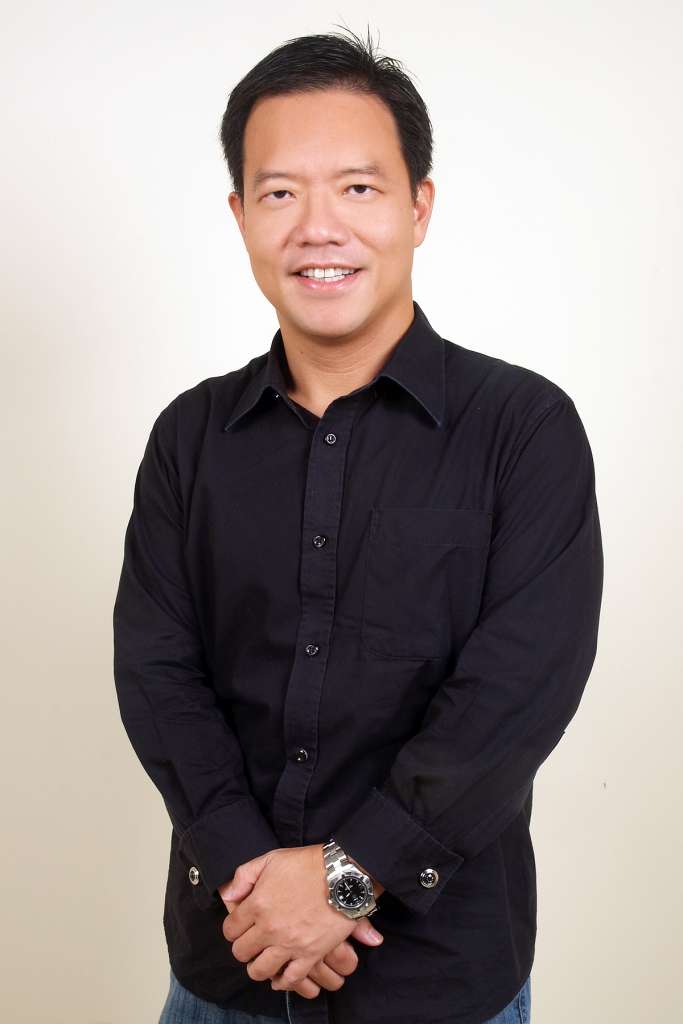
“We tend to think machine learning is in the realm of data scientists, programmers or hackers, but this is not true; it’s actually something quite simple,” says Huo. “Anyone who spends a bit of time will be able to grasp the fundamentals of artificial and machine learning.”
According to him, AI’s merits lie in its ability to classify items while learning from past inputs and adjusting to new inputs in its replication of “human-like tasks”. Given time, AI can improve its pattern recognition and ultimately complete these tasks with greater efficiency.
Beyond rudimentary processes is the machine learning subset of AI that AAM is optimising for application in financial portfolios. In the near future, AAM will venture further into deep learning, a component of AI that is “more complicated” but “even more accurate” in its predictive ability, Huo claims.
Huo believes that the time spent understanding these systems will be of great benefit to portfolio management. However, he also warns that mismanaged expectations can lead AI algorithms to fail.
Huo, citing a report from Forbes, notes that the project failure rate of AI projects is a staggering 85%. Within this minor proportion of successful AI projects, those belonging to specialised genres such as finance make up an even small number.
“If you put stock market information, market indices, company finances, macroeconomic indicators — everything and the kitchen sink — into this magic machine called AI, expecting it to pick out the top 10 performing stocks, this will fail. You are asking AI to do more than it possibly can,” he explains, pointing out that financial data contains “too much noise”.
Given that humans are unable to fully predict market movements to pick out good stocks, Huo says that AI cannot be expected to simply be able to pick out the best-performing stocks. Rather, AAM’s machine learning has a more reasonable task for AI, where data is used in a way not dissimilar from what would be used by human analysts. Only 50 each of fundamental, technical and financial journal indicators are included in AAM’s feature engineering phase, reducing the input of less relevant data that would cause it to fail.
Explaining the inclusion of financial journal indicators, Huo says that AAM is “agnostic” in that regard, and that excluding the “wisdom” of researchers who have spent decades studying particular phenomena would not be sensible for their machine learning.
Using this data, machine learning can rank individual stocks in a group, based on each stock’s probability of falling above or below the median line in this group. This ranking allows machine learning to pick more stocks from above the 50th percentile and fewer from those that fall below the threshold.
AAM puts its data and processes through what it claims to be the “most rigorous” form of back testing known as “walk forward” tests, in which output from historical data is compared with actual returns. For example, the machine learning’s predictive output based on data from 2007 to 2019 is compared with actual results from 2020 to determine its accuracy.
Returning to Kong’s emphasis on global diversification, Huo says that AAM’s testing is applied to 16 markets of countries and regions that includes China, Hong Kong, the US and Europe. “We want to test our machine learning to the point of failure. Each country has its own stock market characteristics, so testing Singapore’s market alone is not sufficient,” says Huo.
Proprietary discoveries
This tedious process of verification appears to be working. Stock picks from the first decile (top 10%) of AAM’s machine learning ranking across 10 markets outperformed average returns for the same markets by 11.7% to 5.6% on average for the period of 2008 to 2021.
Huo includes the caveat that this sample comparison is over 13 years and does not guarantee average outperformance for every year. However, he adds that multi-country diversification remains AAM’s defensive “shield” and that AAM is “confident” of delivering results with time.
In the process of its “pioneering work”, AAM has also made proprietary discoveries of indicator groupings that are particularly “impactful” on stock value, says Huo who emphasised the importance of this factor.
AAM’s “biggest discovery”, according to Huo, happened when it found that R&D expenses as a function of market capitalisation combined with two separate indicators — or what AAM has termed “cluster functionalities” — had an impact on share prices.
Huo says that the mathematics and science behind its machine learning algorithms, and what led to the discovery of cluster functionalities are precisely what makes AAM “unique”, despite some of these strategies seeming “obvious”. “We still need time and diversification for this to play out, but we are confident that over time we will win,” he says.
“Our investment methodology, the philosophy of AAM has not changed. AI merely does the same tasks that a human analyst would — but it is able to cope with far more variables. Our fund managers add a human layer when they filter out stocks deemed high risk from the portfolio,” says Huo. “Diversification has not been abandoned. There is very little over-concentration risk in AAM.”
Huo reiterates that machine learning exists as part of AAM’s process to “enhance” but not replace its investment strategy, and adds that there is more work to be done to improve its model and analytical abilities — an endeavour perhaps all the more pertinent in periods of market volatility, such as now. “For investors, this is really the time to seek investment, not to run in fear,” he says.
Reproduced from The Edge Singapore
Group picture: From left, Harry Huo, Eric Kong and Prof Kishore Mahbubani of Aggregate Asset Management. They were all speakers at a recent forum moderated by Goola Warden of The Edge Singapore.